In today’s competitive job market, organizations are increasingly recognizing the need to adopt data-driven approaches to optimize their hiring practices. Talent acquisition has evolved from a subjective and intuition-based process to one where empirical evidence and experimentation play a pivotal role. This shift has been powered by the application of A/B testing—a method borrowed from the world of digital marketing and product development—to the realm of recruitment.
This guide, “How to Optimize Hiring Practices through A/B Testing in Recruitment with Data-Driven Experimentation,” delves into the transformative potential of data-driven experimentation in the hiring process. A/B testing, a technique known for its ability to compare two or more variants and determine which yields superior results, has found its place in recruitment to enhance decision-making, streamline processes, and ultimately find the best-fit candidates.
As we explore the intricacies of A/B testing in the context of recruitment, this guide will provide practical insights, case studies, and expert advice to empower organizations to leverage the power of experimentation in their hiring strategies. Whether you are a seasoned HR professional or new to the concept of data-driven hiring, this guide will equip you with the knowledge and tools to make more informed, efficient, and successful hiring decisions.
Hirebee ensures that you are able to find the best candidates in the market for your team through industry-leading candidate screening as well as candidate sourcing features.
Table of Contents
ToggleUnderstanding A/B Testing in Recruitment
A/B testing, also known as split testing, is a powerful methodology employed in various industries to optimize and enhance decision-making processes. In the realm of recruitment, it offers a data-driven approach to transform traditional hiring practices into more efficient and effective systems.
At its core, A/B testing involves comparing two or more variations of a specific element or process to determine which one performs better in achieving predefined objectives. In the context of recruitment, this means experimenting with different strategies, tools, or approaches to identify the most successful ones in attracting, screening, and hiring candidates.
The benefits of A/B testing in recruitment are multifaceted. First and foremost, it provides tangible insights into what works and what doesn’t, enabling organizations to allocate resources more wisely. By systematically testing different aspects of their recruitment process, companies can refine their strategies, reduce time-to-hire, lower recruitment costs, and ultimately secure top talent more efficiently.
Key metrics and key performance indicators (KPIs) play a pivotal role in A/B testing. They allow recruiters to measure the impact of changes accurately, ensuring that the experimentation process is both meaningful and informed. Examples of relevant metrics include applicant conversion rates, time-to-fill, candidate quality, and diversity in applicant pools.
In this section, we will delve deeper into the concept of A/B testing in recruitment, emphasizing its advantages and the metrics that matter most in the quest to optimize hiring practices. Understanding these fundamentals is essential before embarking on the journey of data-driven experimentation in talent acquisition.
Preparing for A/B Testing in Recruitment
Before embarking on the journey of optimizing hiring practices through A/B testing, careful preparation is essential. This stage lays the foundation for effective experimentation and ensures that your efforts yield valuable insights.
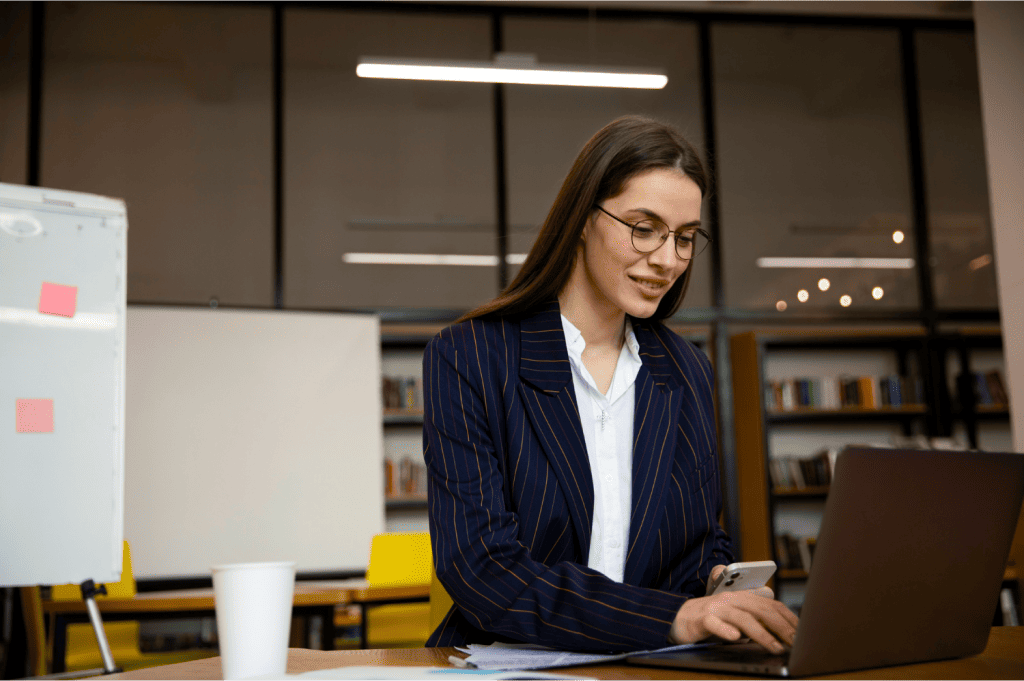
Here’s how to prepare for A/B testing in recruitment:
Identifying the areas for experimentation:
To begin, pinpoint the specific aspects of your recruitment process that require improvement. This could encompass job postings, candidate sourcing strategies, screening processes, or interview techniques. Identifying these areas helps you focus your efforts on what matters most and increases the likelihood of impactful results.
Defining clear objectives and hypotheses:
Every A/B test should have a well-defined purpose and hypothesis. Clearly articulate what you aim to achieve through the experiment. For example, you may want to test whether modifying the language in job postings leads to higher-quality applicants. Having a precise objective and hypothesis ensures you measure the right variables and evaluate success effectively.
Building a diverse talent pool for testing:
To ensure your A/B tests produce meaningful results, it’s crucial to have a diverse pool of candidates. This diversity should encompass factors like skills, experience, and demographics. A varied candidate pool helps in evaluating the impact of changes on different segments of potential hires and ensures the findings are applicable to a broader range of recruitment scenarios.
Effective preparation in these areas sets the stage for successful A/B testing in recruitment, helping organizations make data-driven decisions and continually improve their hiring practices.
Setting Up A/B Experiments
Setting up A/B experiments in recruitment is a critical phase in your journey toward optimizing hiring practices through data-driven experimentation. This phase involves translating your objectives and hypotheses into actionable tests that can yield meaningful insights for improvement. Here’s a closer look at the key aspects of this process:
Formulating the control and experimental groups
Start by defining two distinct groups, the control group and the experimental group. The control group represents your existing hiring practices or the status quo, while the experimental group incorporates the changes or improvements you want to test. Ensure that these groups are comparable in terms of relevant factors such as job roles, experience levels, and demographics to obtain accurate results.
Selecting the right variables to test
Choose specific variables or factors within the recruitment process that you intend to test. These variables can range from alterations in job descriptions, changes in candidate outreach strategies, modifications in screening questions, to adjustments in interview formats. The selection of variables should align with your objectives and hypotheses.
Designing the A/B testing plan
Create a detailed plan that outlines how you will execute the A/B tests. Define the duration of the experiments, the sample sizes for both groups, and the criteria for success. A well-structured plan helps ensure that the experiments are conducted consistently and that the results are reliable.
Allocating resources and budget
Adequate resources, including time, personnel, and budget, are essential for the successful execution of A/B experiments. Allocate resources judiciously to gather sufficient data and make meaningful conclusions.
Ensuring legal and ethical compliance
Before proceeding with your A/B tests, ensure that your experiments comply with all legal and ethical standards. Respect candidates’ privacy, avoid discriminatory practices, and adhere to data protection regulations such as GDPR or HIPAA, depending on your jurisdiction.
The process of setting up A/B experiments requires careful planning and attention to detail, but it forms the foundation for data-driven decision-making in recruitment, allowing you to uncover insights that can lead to more effective hiring practices.
Implementing A/B Tests
Implementing A/B tests is a critical phase in optimizing hiring practices through data-driven experimentation. This stage involves putting your well-planned experiments into action and gathering the necessary data to assess their impact.
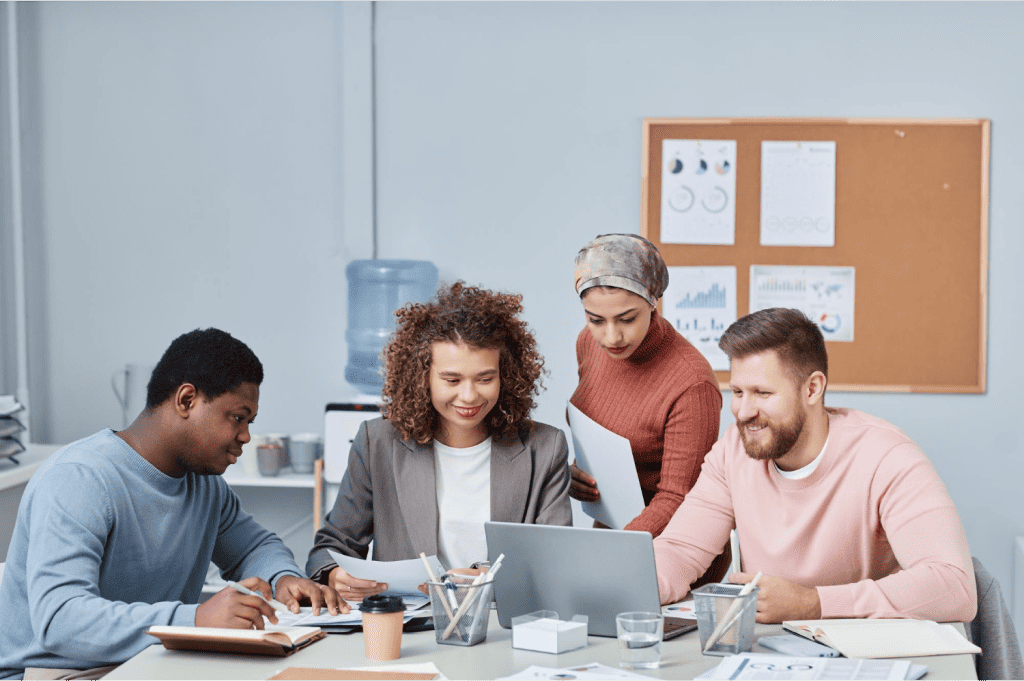
Here’s a breakdown of key considerations for this stage:
Executing the Experiments
Start by implementing the A/B tests in real-world recruitment scenarios. For instance, if you are testing different job descriptions, ensure that each version is used for actual job postings. Maintain consistency in how candidates are sourced, screened, and interviewed to isolate the impact of the specific changes being tested.
Tracking and Data Collection
Rigorous data collection is essential. Utilize applicant tracking systems, HR analytics platform, and surveys to gather relevant data. Ensure that you capture both quantitative metrics (e.g., application rates, interview conversion rates) and qualitative feedback from candidates and hiring teams.
Maintaining Consistency
Throughout the testing phase, it’s vital to maintain consistency in processes and procedures. Any deviation from the plan can introduce bias and skew results. Ensure that all team members involved are aware of the ongoing experiments and adhere to the established protocols.
Troubleshooting and Adapting
Be prepared to address any unexpected challenges or issues that may arise during the testing phase. Regularly monitor the experiments, and if necessary, make adjustments to ensure the validity of the results.
Implementing A/B tests effectively demands careful execution and attention to detail. By following this phase diligently, you can gather robust data that will guide your decisions in refining your hiring practices.
Analyzing A/B Test Results
Analyzing A/B test results is a critical step in optimizing hiring practices through data-driven experimentation. This phase involves a systematic evaluation of the outcomes of your experiments to make informed decisions. Here’s how you can effectively analyze your A/B test results:
Statistical Significance and Interpretation of Results:
- Begin by assessing the statistical significance of your results. Statistical significance helps determine if the observed differences between the control and experimental groups are likely due to the changes you implemented or if they could be attributed to chance.
- Use appropriate statistical tests, such as t-tests or chi-squared tests, to calculate p-values and confidence intervals.
- Interpret the results in the context of your original objectives and hypotheses. Are the changes you made leading to meaningful improvements in your recruitment metrics? Are they aligned with your goals?
Identifying Patterns and Trends:
- Look for patterns and trends in your data. Are there consistent patterns of improvement or decline across multiple experiments?
- Segment your data to understand how different subgroups of candidates or job roles may be impacted differently.
- Consider conducting post-experiment surveys or interviews with candidates and hiring managers to gather qualitative insights that can complement quantitative data.
Assessing the Impact on Recruitment Metrics:
- Evaluate the impact of your A/B tests on key recruitment metrics, such as time-to-fill, cost-per-hire, and quality-of-hire.
- Compare the performance of the control and experimental groups over time to assess the sustainability of the changes.
- Determine if any unexpected consequences or side effects have emerged as a result of your experiments.
Making Data-Driven Decisions Based on Results:
- Based on the analysis of your A/B test results, make informed decisions about whether to adopt, modify, or discard the changes implemented during the experiments.
- Communicate the findings and decisions to relevant stakeholders and ensure transparency in the decision-making process.
- Use the insights gained to iterate and refine your hiring practices, setting the stage for ongoing optimization.
Effective analysis of A/B test results empowers organizations to refine their hiring processes, ultimately leading to more efficient and successful recruitment practices.
Iterative Optimization
The journey toward optimizing hiring practices through A/B testing doesn’t conclude with the successful completion of experiments; it’s an ongoing process of refinement and improvement. Iterative optimization is a crucial phase where organizations build on the insights gained from A/B tests and consistently evolve their recruitment strategies.
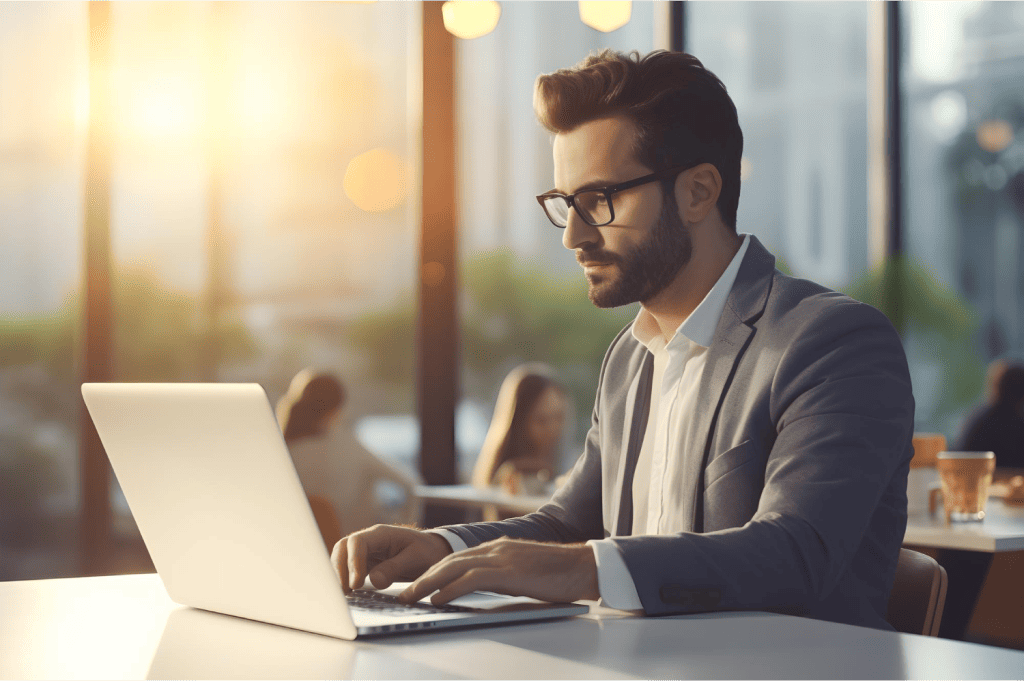
Incorporating successful changes
Once A/B tests identify improvements, these changes should be seamlessly integrated into the standard hiring processes. This involves updating job postings, modifying interview techniques, or adjusting candidate sourcing methods based on the data-backed findings.
Continuously refining and experimenting
Recruitment is dynamic, and what works today might not work tomorrow. Therefore, it’s essential to continue experimenting and adapting to changing circumstances. Regularly reassessing and fine-tuning the A/B testing plan ensures that hiring practices remain effective and competitive.
Measuring long-term effects
The impact of A/B test-driven changes should be assessed over time. Organizations must monitor recruitment metrics over extended periods to determine the sustainability of improvements and to identify any unforeseen consequences.
Iterative optimization is not a one-time effort but a commitment to ongoing enhancement of recruitment processes. By embracing this approach, organizations can stay ahead in the competitive talent landscape and consistently attract top candidates while minimizing biases and inefficiencies.
Conclusion
In conclusion, leveraging A/B testing in recruitment through data-driven experimentation is a transformative approach that empowers organizations to make smarter, more effective hiring decisions. By systematically testing and optimizing various aspects of the hiring process, businesses can improve candidate experiences, reduce bias, and enhance overall talent acquisition outcomes. This approach fosters a culture of continuous improvement, ensuring that hiring practices remain adaptive and competitive. As the job market evolves and candidate expectations change, embracing A/B testing becomes not only a best practice but a strategic necessity for organizations seeking to attract and retain top talent. It’s a path forward towards a more efficient, equitable, and successful recruitment process.
Hirebee makes sure that you are able to make the most of your recruiting efforts, as you will no longer have to worry job distribution or reporting and analytics.
FAQs
What is A/B testing in the context of recruitment?
A/B testing in recruitment involves comparing two or more variations of a hiring process to determine which one performs better in terms of attracting, evaluating, and selecting candidates.
Why should my organization consider A/B testing for recruitment?
A/B testing helps organizations make data-driven decisions, reduce biases in hiring, improve the candidate experience, and ultimately hire more qualified candidates.
What are some areas within recruitment that can be optimized using A/B testing?
You can experiment with job postings, candidate sourcing methods, screening processes, interview techniques, and even candidate communication to improve your recruitment outcomes.
How do I get started with A/B testing in recruitment?
Begin by identifying areas for experimentation, formulating clear objectives, and designing a testing plan. Ensure you have the necessary resources and a compliant process.
What are some common metrics and KPIs used in A/B testing for recruitment?
Metrics might include application conversion rates, time to hire, cost per hire, and candidate satisfaction scores, among others.