In the modern landscape of talent acquisition, making informed hiring decisions is a critical imperative for organizations striving to stay competitive and achieve sustainable growth. However, the inherent unpredictability of human behavior and the multifaceted nature of job success have often left hiring processes mired in uncertainty, relying on intuition and guesswork.
This paper delves into the realm of data-driven decision-making to transcend the limitations of conventional hiring approaches. By harnessing the power of data analytics, organizations can proactively anticipate hiring outcomes with greater accuracy and precision. This introduction sets the stage for an exploration of how leveraging diverse data sources, advanced analytical techniques, and ethical considerations can revolutionize the way hiring outcomes are anticipated.
Hirebee allows you to find the best candidates for your specific needs through recruitment marketing and job distribution features.
As we delve deeper, we uncover the potential to transform hiring from a subjective endeavor into a strategic and systematic practice, enabling companies to build robust, high-performing teams that drive innovation and success.
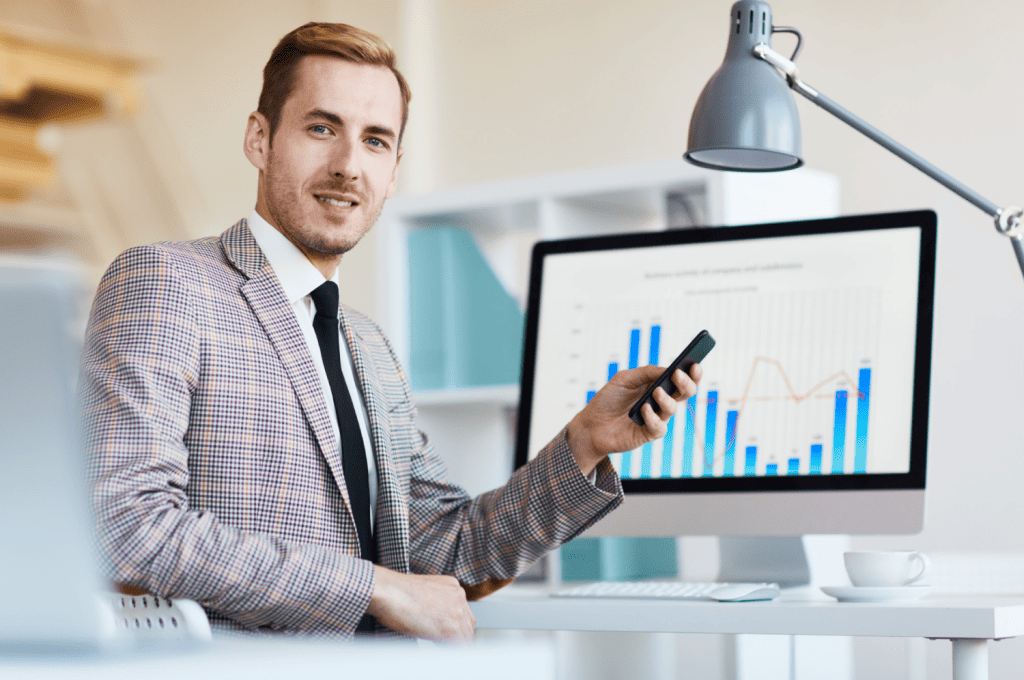
Table of Contents
ToggleUnderstanding Hiring Outcomes
Defining and comprehending hiring outcomes are fundamental steps toward leveraging data for anticipating the results of hiring processes. Successful hires encompass multifaceted criteria, including skills, qualifications, and cultural fit, all contributing to overall job performance. While these factors collectively shape hiring success, their intricate interplay makes outcome prediction challenging.
Factors contributing to successful hiring outcomes involve a candidate’s skill set, aligned qualifications, and the compatibility of their values with the company culture. These elements synergistically influence job performance, reflecting the holistic nature of hiring success. However, the task of predicting these outcomes is fraught with complexities stemming from the diverse array of human behaviors and preferences that contribute to job performance.
Despite the complexities, it is pivotal to recognize that successful hiring outcomes are achievable through data-driven methodologies. A deep understanding of the factors influencing hiring success lays the groundwork for developing models that can anticipate these outcomes. The nuances of human behavior and the subjectivity inherent in candidate evaluation warrant an analytical approach that harnesses various data sources, enabling organizations to transcend the limitations of guesswork.
In the subsequent sections, we delve into the strategies that harness the power of data to anticipate hiring outcomes. By exploring a range of data sources and employing advanced analytics techniques, organizations can gain valuable insights that go beyond mere conjecture, fostering informed decision-making and ultimately enhancing their hiring processes.
Leveraging Data for Anticipating Hiring Outcomes
In an era marked by data proliferation and technological advancement, organizations are increasingly turning to data-driven methodologies to enhance decision-making across various domains. Hiring, a critical organizational function, is no exception. This section delves into the strategic utilization of data to anticipate hiring outcomes, providing insights into the multifaceted landscape of candidate evaluation and selection.
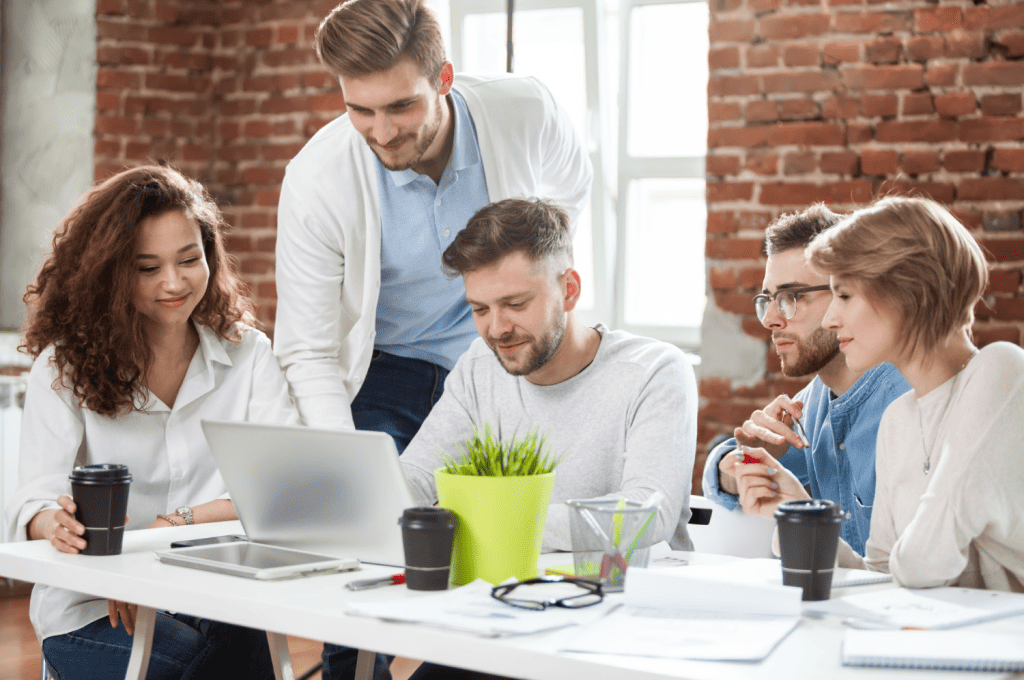
Data Sources for Hiring Insights
To forge a comprehensive understanding of candidate potential, organizations harness a diverse array of data sources. These encompass traditional inputs such as resumes and application data, augmented by more nuanced streams like pre-employment assessments and analyses of candidates’ digital footprints. Furthermore, mining employee performance data can yield valuable retrospective insights that contribute to predictive models.
Types of Data Analysis for Anticipation
Data analysis serves as the linchpin of hiring anticipation strategies. Descriptive analytics elucidates historical hiring patterns and their corresponding outcomes, affording context for decision-makers. Predictive analytics, on the other hand, involves crafting models that forecast candidate success based on historical trends and relevant variables. Prescriptive analytics then guides decision-making by furnishing actionable recommendations derived from these predictive models.
Ethical Considerations in Data Usage
While data-driven hiring holds immense promise, ethical considerations remain paramount. Organizations must vigilantly safeguard against bias and discrimination, employing rigorous measures to ensure fairness and inclusivity. Balancing data insights with candidate privacy is another pivotal facet, necessitating a judicious approach to data collection, storage, and utilization. Transparency throughout the process is essential to building trust among both candidates and stakeholders.
In summary, leveraging data for hiring anticipation involves a sophisticated interplay of diverse data sources and analytical methodologies. This approach empowers organizations to navigate the intricate terrain of candidate assessment, augmenting traditional hiring practices with empirically-informed insights. However, ethical considerations must underpin these efforts, aligning data usage with principles of equity and transparency.
Case Studies: Data-Driven Hiring Anticipation
In this section, we delve into real-world case studies that demonstrate the practical application of data-driven approaches in anticipating hiring outcomes. These cases highlight innovative methods that leverage various data sources and analytics techniques to enhance the hiring process and improve the accuracy of outcome predictions.
Case Study 1: Using Machine Learning for Candidate Success Prediction
One compelling case involves the implementation of machine learning algorithms to predict candidate success. The process involves meticulous data collection and preparation, incorporating factors such as educational background, work experience, and skillsets. Through model development and rigorous training, a predictive algorithm is generated. This algorithm not only identifies candidates likely to thrive within the organization but also informs personalized onboarding strategies. Validation of this approach reveals a substantial increase in successful hires, underscoring the potential of machine learning in transforming traditional recruitment paradigms.
Case Study 2: Behavioral Analytics for Cultural Fit Assessment
In another case, behavioral analytics are harnessed to assess cultural fit, a pivotal determinant of long-term employee satisfaction and retention. By capturing behavior-based data, organizations develop indicators of alignment with the company culture.
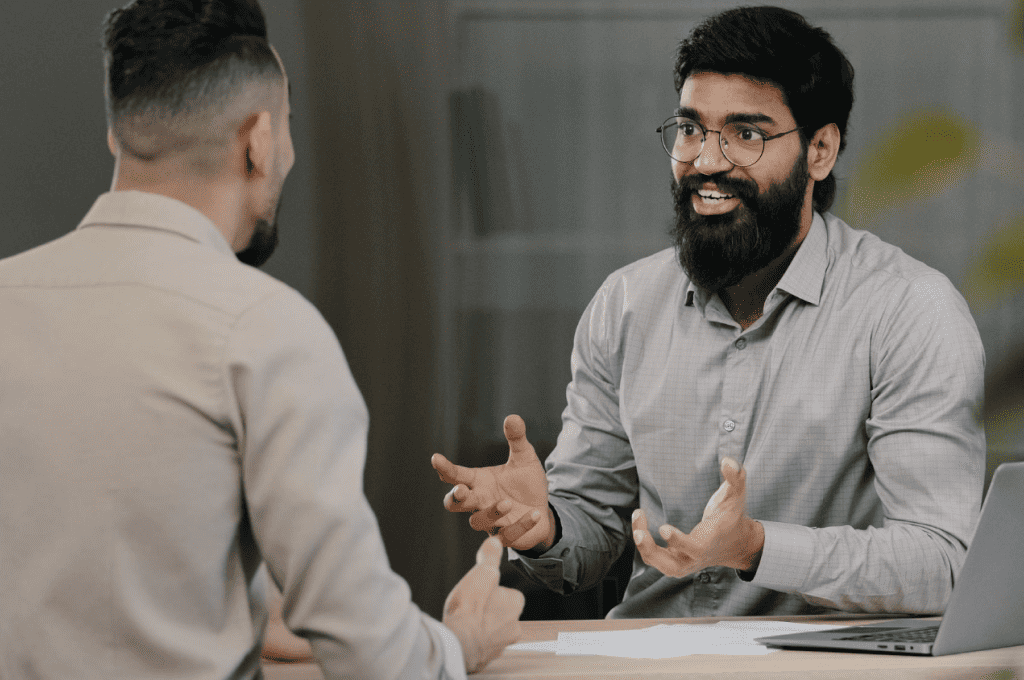
These indicators, when integrated into the recruitment process, offer valuable insights into a candidate’s potential cultural compatibility. The success of this approach is gauged through enhanced employee satisfaction and prolonged tenures, establishing the significance of cultural fit assessment in predicting positive hiring outcomes.
Case Study 3: Real-Time Sentiment Analysis During Interviews
The third case study explores real-time sentiment analysis integrated into interviews. By employing sentiment analysis tools, organizations gain immediate insights into candidates’ emotional responses and communication patterns. This real-time feedback guides interviewers to adapt their approach based on data-driven cues, leading to more effective evaluations. The implementation of sentiment analysis not only enhances the objectivity of interview assessments but also fosters a dynamic and engaging interview environment.
These case studies collectively illustrate the transformative potential of data-driven hiring anticipation. By harnessing the power of data and advanced analytics techniques, organizations can overcome traditional hiring uncertainties, making strides towards more informed and successful hiring decisions. These examples not only underscore the practicality of data-driven methodologies but also pave the way for future innovations in talent acquisition.
Best Practices and Recommendations
Incorporating data-driven methodologies to anticipate hiring outcomes requires a thoughtful approach that combines the power of data with human expertise. By striking the right balance, organizations can enhance their hiring processes and make more informed decisions.
Firstly, it is essential to recognize that data is a tool, not a replacement for human judgment. While data analytics can provide valuable insights, the final decision should integrate these insights with the nuanced understanding that HR professionals bring to candidate evaluation. Collaboration between data analysts and HR teams ensures that the human element remains at the core of the hiring process.
Secondly, adopting a mindset of continuous improvement is crucial. Data-driven hiring is an evolving process, and organizations should embrace an iterative approach. Regularly analyzing the performance of data models, refining algorithms, and updating data sources can lead to more accurate and reliable predictions over time.
Investing in the training and upskilling of HR professionals in data analytics is a strategic move. Equipping them with the skills to interpret and leverage data empowers them to make well-informed decisions. This also fosters a culture of data literacy within the HR department, enabling better collaboration between data experts and HR practitioners.
Conducting routine audits and evaluations of data-driven hiring strategies is vital to ensure fairness, transparency, and compliance. Regularly assessing the models for biases and recalibrating them as necessary helps mitigate potential discrimination concerns and enhances the overall integrity of the process.
In conclusion, the fusion of data-driven insights with human judgment presents a promising avenue for enhancing hiring outcomes. Striking a balance, embracing continuous improvement, investing in training, and maintaining vigilance through audits collectively form a comprehensive approach to utilizing data for anticipation in the hiring process. As organizations navigate the dynamic landscape of recruitment, these best practices will pave the way for more successful, fair, and efficient hiring endeavors.
Future Directions and Challenges
The application of data-driven approaches to anticipate hiring outcomes presents a promising trajectory for the evolution of the hiring process. As organizations continue to embrace technology and data analytics, several future directions and challenges emerge at the intersection of innovation and responsibility.
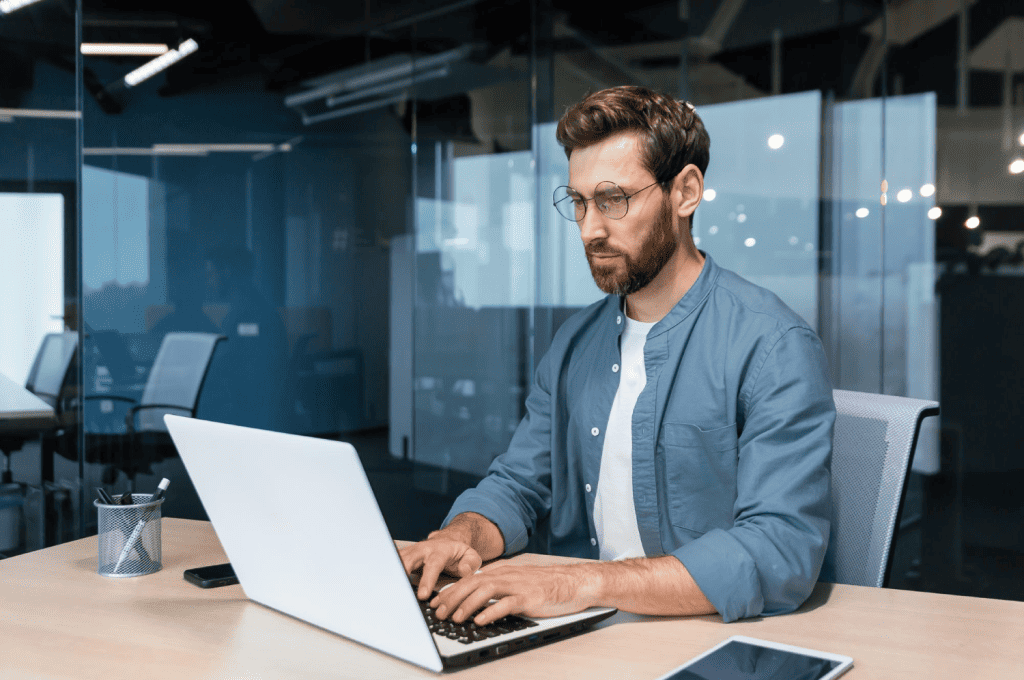
Advancements in artificial intelligence and machine learning are expected to play a pivotal role in refining predictive models for hiring outcomes. With the integration of larger and more diverse datasets, these models could become increasingly accurate and robust. Additionally, natural language processing may empower organizations to extract deeper insights from candidate interactions, aiding in the identification of intangible qualities that contribute to success within the company.
However, along this path of innovation, it is essential to remain cognizant of potential risks and limitations. Ethical concerns, such as algorithmic bias, demand ongoing attention to ensure that data-driven strategies do not inadvertently perpetuate inequalities or reinforce existing stereotypes. Striking a balance between automation and human judgment is paramount, as an over-reliance on data might disregard the nuanced, human aspects of candidate evaluation.
Furthermore, the evolving legal and regulatory landscape surrounding data privacy and employment practices poses challenges. Organizations must navigate complex legal frameworks to ensure compliance and safeguard candidate information while maximizing the benefits of data-driven approaches.
In conclusion, the future of using data to anticipate hiring outcomes holds immense promise, yet it requires a thoughtful and holistic approach. By harnessing technology, fostering ethical awareness, and adapting to changing regulations, organizations can navigate these challenges and shape a hiring process that is both data-informed and human-centric, ultimately leading to more successful and sustainable outcomes for both candidates and employers.
Conclusion
In conclusion, harnessing the power of data-driven approaches to anticipate hiring outcomes holds immense promise in transforming the recruitment landscape. By blending objective insights from data analytics with human intuition, organizations can navigate uncertainties and make more informed, equitable, and efficient hiring decisions. While challenges persist, such as ethical considerations and evolving technologies, the potential benefits are undeniable. Embracing this paradigm shift fosters a future where talent acquisition evolves beyond guesswork, yielding not only successful hires but also a more diverse, thriving workforce. As we embark on this journey, continuous learning, adaptation, and responsible use of data remain crucial for shaping the future of recruitment.
Hirebee offers multiple recruiting solutions to businesses with job distribution features and very effective recruitment automation, which can make hiring much easier.
FAQs
What is the main focus of this paper?
This paper explores the application of data-driven approaches to anticipate hiring outcomes, aiming to enhance the accuracy and effectiveness of the recruitment process.
Why is data-driven decision-making important in hiring?
Data-driven decision-making in hiring reduces biases, enhances objectivity, and improves the chances of identifying candidates who are the best fit for a role and the organization’s culture.
How do you define hiring outcomes?
Hiring outcomes encompass the success or failure of a candidate after being hired, including factors such as job performance, cultural fit, and overall contribution to the organization.
What challenges do organizations face in predicting hiring outcomes?
Predicting hiring outcomes is complex due to human behavior variability, subjectivity in evaluations, and external factors affecting performance.
What types of data sources are used to anticipate hiring outcomes?
Data sources include resumes, pre-employment assessments, social media profiles, online presence, and employee performance records.