In today’s fast-paced and competitive business environment, the role of Human Resources (HR) is undergoing a significant transformation. Traditionally seen as a support function, HR is now emerging as a strategic powerhouse within organizations. With increasing pressure to attract, develop, and retain top talent, HR is evolving to take a more proactive approach to decision-making. The days of relying solely on intuition and historical practices are fading, as organizations seek more efficient, data-driven solutions to workforce challenges.
Predictive analytics is at the forefront of this transformation, enabling HR professionals to move from reactive problem-solving to proactive talent management. By leveraging advanced data analysis, machine learning, and artificial intelligence (AI), predictive analytics allows HR teams to identify patterns, anticipate trends, and forecast future workforce needs with greater accuracy. Instead of relying solely on past performance or instinct, HR departments can now use vast amounts of data to predict crucial outcomes such as employee performance, turnover, talent gaps, and more. This shift not only improves the efficiency of HR processes but also positions HR as a strategic partner that drives business outcomes and organizational success.
Table of Contents
ToggleWhat Is Predictive Analytics for HR?
Predictive analytics for HR is a game-changing approach that uses advanced data analysis techniques, artificial intelligence (AI), and machine learning algorithms to predict future workforce trends, behaviors, and outcomes. It moves beyond traditional methods by not just looking at historical data or current employee performance but analyzing a wide range of variables to forecast what will likely happen in the future. This foresight enables HR teams to make proactive, data-driven decisions that align with the company’s long-term goals.
At its core, predictive analytics aggregates and analyzes data from multiple sources, such as employee demographics, performance reviews, recruitment efforts, engagement surveys, and turnover rates. By recognizing hidden patterns and trends, it helps HR anticipate challenges—like potential employee attrition or gaps in skill sets—and take action before these challenges escalate. With the insights provided by predictive analytics, HR leaders can implement targeted retention strategies, adjust recruitment efforts, or align talent management plans with evolving business needs. This forward-thinking approach helps HR move from a reactive function to one that strategically drives organizational growth, ensuring a competitive advantage in talent management and business success.
Key Benefits of Predictive Analytics in HR
Predictive analytics is revolutionizing HR by shifting the function from a reactive to a proactive, strategic role. Here’s how predictive analytics is driving meaningful change in HR.
Enhanced Talent Acquisition and Retention
Hiring the right talent is critical to business success, but traditional recruitment methods often rely on subjective assessments, leading to mismatches and higher turnover rates. Predictive analytics helps HR teams refine their hiring strategies by analyzing historical hiring data, candidate attributes, and performance trends to identify the best predictors of long-term success.
- Better candidate selection: AI-powered models assess resumes, interview performance, and assessment results to determine which candidates have the highest potential for success.
- Reducing turnover risks: Predictive models analyze factors contributing to employee departures, allowing HR to implement targeted retention strategies, such as competitive compensation, career development programs, and improved workplace culture.
- Optimizing hiring channels: By evaluating past recruitment efforts, HR can determine which sources yield the highest-quality candidates and focus resources accordingly.
Optimized Workforce Planning
Workforce demand fluctuates due to market conditions, business growth, and industry trends. Without accurate forecasting, organizations risk understaffing or overstaffing, both of which can negatively impact productivity and costs. Predictive analytics enables HR to plan by anticipating workforce needs based on historical data and business projections.
- Demand forecasting: HR can anticipate hiring needs by analyzing trends in employee turnover, retirement patterns, and business expansion plans.
- Skill gap analysis: Predictive models identify upcoming skills shortages, helping HR design targeted training programs or adjust recruitment strategies.
- Cost efficiency: By ensuring the right number of employees are in place, organizations can avoid unnecessary hiring costs and minimize productivity losses.
Proactive Employee Engagement Strategies
Employee engagement directly impacts productivity, job satisfaction, and retention. Traditional engagement strategies rely on periodic surveys and reactive interventions, which may not address underlying issues in time. Predictive analytics enables HR to assess engagement levels in real-time and implement proactive measures to improve workplace morale.
- Identifying disengagement trends: By analyzing factors such as absenteeism, feedback trends, and productivity levels, HR can detect early signs of disengagement.
- Personalized employee experiences: AI-driven insights help HR develop customized engagement strategies, such as flexible work arrangements, tailored career development paths, and recognition programs.
- Preventing burnout: Predictive analytics can identify workload imbalances and stress indicators, allowing HR to take preventive action, such as adjusting workloads or providing wellness resources.
Data-Driven Performance Management
Traditional performance evaluations often suffer from bias, inconsistency, and lack of real-time insights. Predictive analytics transforms performance management by providing objective, data-driven insights that enhance decision-making and employee development.
- Continuous performance tracking: HR can monitor key performance indicators (KPIs) in real time, allowing for timely feedback and adjustments.
- Personalized development plans: AI-driven models assess strengths and weaknesses, helping HR create tailored training programs that align with individual career goals.
- Objective decision-making: By relying on data rather than subjective assessments, HR ensures fair performance evaluations and promotions based on merit and potential.
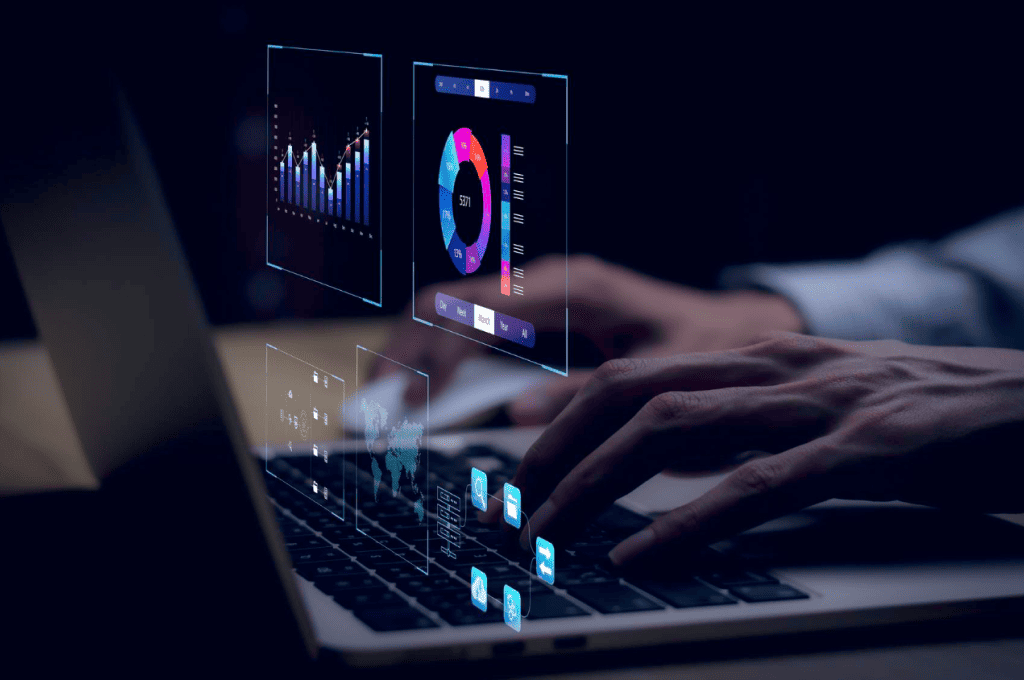
How to Build Effective Predictive Analytics Models in HR
A well-structured predictive analytics model helps organizations forecast trends, mitigate risks, and implement proactive strategies. Below are the key steps to building an effective predictive analytics model for HR.
Step 1: Define HR Metrics and Objectives
Start by setting clear objectives that align with organizational goals. These objectives will guide which HR metrics to focus on, such as employee turnover, recruitment efficiency, employee engagement, and workforce planning. Identifying the right metrics ensures the model provides valuable insights for decision-making.
Step 2: Gather and Preprocess Relevant Data
Data is the backbone of predictive analytics. Collect high-quality data from various sources such as employee records, recruitment data, engagement surveys, and turnover statistics. Preprocessing is crucial to clean the data—handle missing values, remove duplicates, and standardize formats. Visualization tools like heatmaps help identify patterns that inform model development.
Step 3: Select and Implement Predictive Models
Choose the right model based on the HR challenge at hand. Common models include:
- Regression Analysis: Predicts numerical outcomes (e.g., attrition rates or salary trends).
- Classification Models: Categorizes employees by performance or likelihood of turnover.
- Clustering Algorithms: Segments employees based on engagement levels or career development.
- Time Series Analysis: Forecasts trends such as hiring needs or workforce fluctuations.
These models can be implemented using tools like Python, R, or machine learning platforms like TensorFlow and Scikit-learn.
Step 4: Validate and Refine Models for Accuracy
Once the model is implemented, validate its accuracy by comparing predictions to actual outcomes. Use techniques like cross-validation to measure reliability. Continuously refine the model by fine-tuning parameters and incorporating new data to maintain its relevance and precision. Regular monitoring ensures the model’s effectiveness in guiding data-driven HR decisions.
Analyzing and Interpreting HR Data for Predictive Insights
Data analysis and interpretation are crucial components of predictive analytics for HR, as they provide meaningful insights that guide decision-making processes. In this phase, HR professionals leverage various analytical techniques to make sense of the collected data and extract valuable knowledge. There are four main types of analytics: descriptive, diagnostic, predictive, and prescriptive.
Descriptive analytics involves summarizing historical HR data to gain a comprehensive understanding of past trends and events. This step helps identify patterns, anomalies, and general statistics, laying the foundation for more sophisticated analyses. Diagnostic analytics, on the other hand, delves deeper into the data to explore the reasons behind specific outcomes or trends. Understanding the “why” behind the observed patterns enables HR professionals to address underlying issues effectively.
Predictive analytics takes the analysis to the next level by forecasting future HR trends and potential outcomes based on historical data and statistical models. These predictions provide HR departments with valuable foresight, allowing them to proactively prepare for challenges or capitalize on opportunities.
Finally, prescriptive analytics offers actionable recommendations by simulating different scenarios and assessing the potential impact of various decisions. This empowers HR leaders to make well-informed choices, optimize workforce strategies, and improve overall organizational performance.
Common Challenges in Predictive Workforce Analytics
Predictive analytics’ implementation is not without challenges. Organizations must address these obstacles to ensure accurate predictions, maintain ethical standards, and protect employee data. Below are some of the most pressing challenges in predictive workforce analytics.
- Data Integration and Quality Issues: HR data often comes from multiple sources, leading to inconsistencies in formats and quality. Without proper integration and data cleansing, inaccurate or incomplete data can result in flawed predictions, impacting HR decisions.
- Privacy and Data Security Concerns: Predictive analytics relies on sensitive employee data, raising concerns about compliance with regulations like GDPR and CCPA. Organizations must ensure data protection and transparency, as mishandling can lead to legal consequences and loss of trust.
- Bias in AI Models: Predictive models can unintentionally perpetuate biases from historical HR data, leading to unfair outcomes. Regular audits and diverse training datasets are necessary to minimize bias and ensure fairness in decision-making.
- Resistance to Change: Many HR professionals and employees may resist adopting predictive analytics due to concerns about job displacement or skepticism about the reliability of AI insights. Overcoming this resistance requires effective training and clear communication about how analytics complement human expertise.
- Scalability and Continuous Improvement: As organizations grow and workforce dynamics evolve, predictive models need to be adaptable. Regular updates and feedback loops are essential for maintaining the accuracy and relevance of predictive analytics over time.
Selecting the Right Predictive Models
Choosing the appropriate predictive models is critical for deriving meaningful insights and making informed decisions. Here, we explore some common predictive models and their applications in HR:
Regression Analysis for Employee Performance Prediction: Regression analysis is a fundamental statistical technique used to examine relationships between employee performance and various influencing factors such as job tenure, training programs, engagement levels, and compensation. By analyzing historical data, HR teams can predict future performance trends, identify high-potential employees, and design personalized development programs. For instance, a company might use regression models to determine whether additional training hours correlate with higher productivity, allowing them to allocate resources more effectively.
Classification Models for Employee Attrition Forecasting: Employee turnover is a major concern for HR teams, and classification models such as decision trees, logistic regression, and support vector machines can help predict which employees are at risk of leaving. These models analyze key variables like job satisfaction, compensation, work-life balance, and career progression to identify patterns associated with attrition. By leveraging these insights, organizations can implement proactive retention strategies, such as career pathing initiatives, competitive benefits, or mentorship programs, ultimately reducing costly turnover.
Time Series Analysis for Workforce Demand Planning: Workforce demand planning requires HR to anticipate staffing needs based on historical trends and external factors. Time series analysis is particularly useful for forecasting hiring needs, seasonal workforce fluctuations, and business cycle impacts. By analyzing past employment data alongside economic indicators, market trends, and organizational growth patterns, HR can make data-driven workforce planning decisions. This ensures optimal staffing levels, preventing both labor shortages and excess hiring.
Choosing the Right Predictive HR Analytics Tool for Your Business
Selecting the right predictive analytics tool is crucial for maximizing efficiency and making well-informed workforce decisions. The right tool should align with your organization’s specific HR needs, integrate seamlessly with existing systems, and provide actionable insights. Below are key factors to consider when choosing a predictive HR analytics tool.
- Data Integration and Compatibility: A good predictive analytics tool should integrate easily with your existing HR systems, such as applicant tracking systems (ATS), payroll software, performance management tools, and employee engagement platforms. The ability to pull data from multiple sources ensures comprehensive analysis, enabling HR teams to make well-rounded decisions based on a complete view of workforce trends.
- User-Friendliness and Accessibility: HR professionals are not always data scientists, so the chosen tool should offer an intuitive interface, easy-to-read dashboards, and clear visualizations. A tool with AI-driven recommendations, natural language queries, and customizable reports makes it easier for HR teams to derive insights without requiring advanced technical expertise.
- Predictive Capabilities and Model Customization: Different HR teams have unique challenges, from predicting attrition to optimizing recruitment. The tool should offer a range of predictive models, and clustering techniques, while also allowing customization based on company-specific needs. Tools that adapt and refine predictions over time provide greater long-term value.
- Data Privacy and Security Compliance: Handling employee data comes with ethical and legal responsibilities. The tool must comply with data protection regulations such as GDPR, CCPA, or industry-specific privacy standards. It should also provide strong encryption, access control, and anonymization features to protect sensitive workforce information.
- Scalability and Cost-Effectiveness: As businesses grow, HR analytics tools should scale accordingly. Cloud-based solutions often offer flexible pricing models that allow companies to start with essential features and expand as needed. Organizations should assess the total cost of ownership, including licensing fees, implementation costs, and ongoing support.
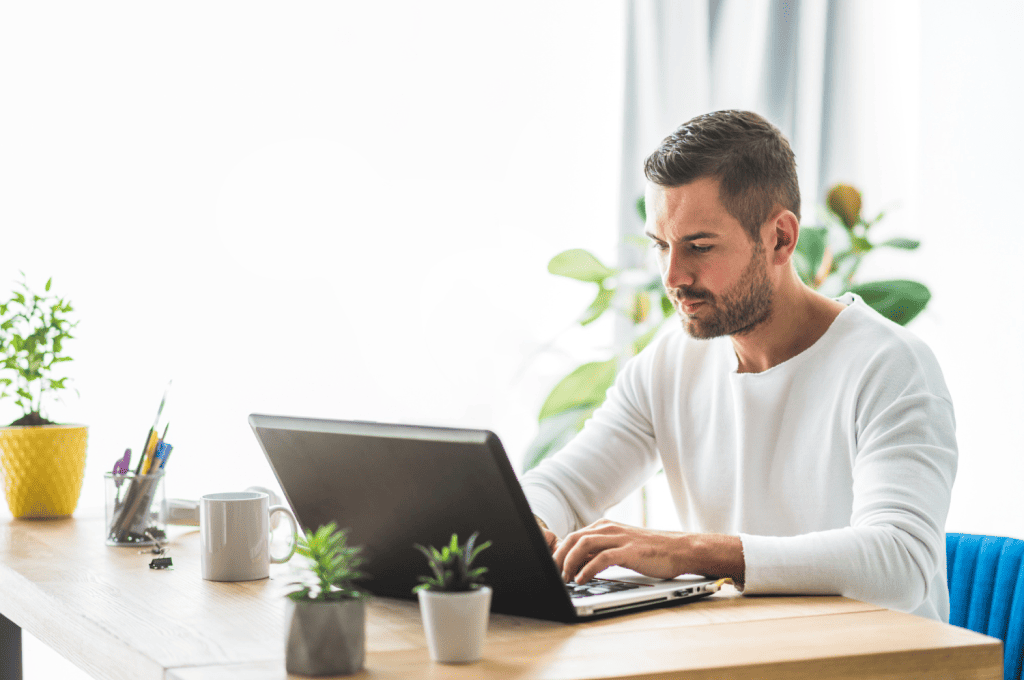
Examples of Predictive Analytics in Action
Below are specific, real-world examples of how organizations leverage predictive analytics to solve common HR challenges, with measurable results.
Reducing Employee Attrition: Hewlett-Packard (HP), with a workforce exceeding 300,000, faced significant turnover challenges, particularly in its sales divisions, where attrition rates reached up to 20%. To combat this, HP developed a predictive model that analyzed factors such as employee performance, span of control, job level, benefits, salary, and supervisor performance. This model enabled HP to identify employees at high risk of leaving and implement targeted retention strategies, effectively reducing turnover rates.
Streamlining Recruitment Processes: Xerox Corporation utilized predictive analytics to enhance its hiring process. By collecting data from personality assessment tests, Xerox identified key personality traits indicative of successful employees. Implementing these insights into their recruitment strategy led to a 20% reduction in attrition within six months, showcasing the efficacy of data-driven hiring practices.
Workforce Demand Forecasting: While specific company examples are limited, predictive analytics has been widely adopted to forecast workforce needs. By analyzing historical data and external factors, organizations can anticipate staffing requirements, optimize recruitment efforts, and reduce costs associated with overstaffing or understaffing.
These cases illustrate the transformative impact of predictive analytics in HR, enabling organizations to make informed decisions that enhance efficiency and employee satisfaction.
Future Trends in HR Predictive Analytics
As technology continues to evolve, the future of HR predictive analytics is poised to become even more sophisticated. Artificial intelligence (AI) and machine learning (ML) are at the forefront of this transformation, driving deeper insights, automation, and real-time decision-making. Below are some key trends shaping the future of HR analytics.
AI-Powered Talent Management and Employee Development: AI is revolutionizing how HR teams manage talent pipelines by providing predictive insights into employee performance and career growth. Machine learning algorithms analyze vast amounts of employee data, uncovering patterns that were previously difficult to detect. For instance, AI can identify skill gaps, suggest personalized learning paths, and predict future leadership potential, allowing HR professionals to proactively foster talent and ensure employees remain competitive in a rapidly changing job market.
Real-Time Predictive Insights for Employee Experience: One of the most exciting advancements in HR analytics is the shift toward real-time data monitoring. Traditional analytics often relied on historical data to inform decisions, but now AI-powered dashboards enable HR teams to track employee sentiment, engagement, and performance in real-time. This allows for immediate intervention, whether to address potential burnout or to improve job satisfaction. By proactively identifying at-risk employees, HR can take targeted actions to reduce attrition and enhance employee retention.
Ethical AI and Bias Mitigation: As predictive analytics continues to integrate into HR processes, addressing ethical concerns becomes paramount. Future HR tools will focus on implementing Explainable AI (XAI) to ensure transparency and fairness in decision-making, particularly around hiring, promotions, and performance evaluations. This trend will help mitigate bias in AI-driven HR decisions, fostering more inclusive, fair, and compliant workplaces while ensuring trust from employees and stakeholders.
Conclusion
Predictive analytics is reshaping HR by offering new opportunities for organizations to enhance decision-making, improve employee engagement, and streamline workforce management. As businesses continue to embrace data-driven strategies, HR teams are becoming better equipped to not only respond to workforce challenges but also anticipate and address them before they arise. With the growing capabilities of AI and machine learning, predictive analytics is moving beyond traditional methods, providing deeper insights that empower HR professionals to make more informed, strategic decisions.
The future of HR is increasingly reliant on advanced analytics to drive continuous improvement. However, organizations must carefully navigate the complexities of data integration, ethics, and model selection to fully leverage the power of predictive analytics. As HR evolves, the ability to harness predictive analytics will be crucial for staying competitive and maintaining a dynamic, engaged workforce.