Employee retention is a critical concern for organizations across industries. In today’s competitive job market, losing valuable talent can have far-reaching consequences, affecting productivity, morale, and the bottom line. Predictive analytics has emerged as a powerful tool to address this challenge, offering a proactive approach to forecast and prevent employee turnover. This document delves into the world of Predictive Analytics for Employee Retention, providing insights into its significance, methodologies, and best practices.
As organizations grapple with the complexities of talent management, the need for data-driven solutions has never been greater. Predictive analytics leverages historical and real-time data to identify potential churn risks, enabling HR professionals to devise targeted strategies for retention. This not only saves recruitment costs but also fosters a more stable and engaged workforce.
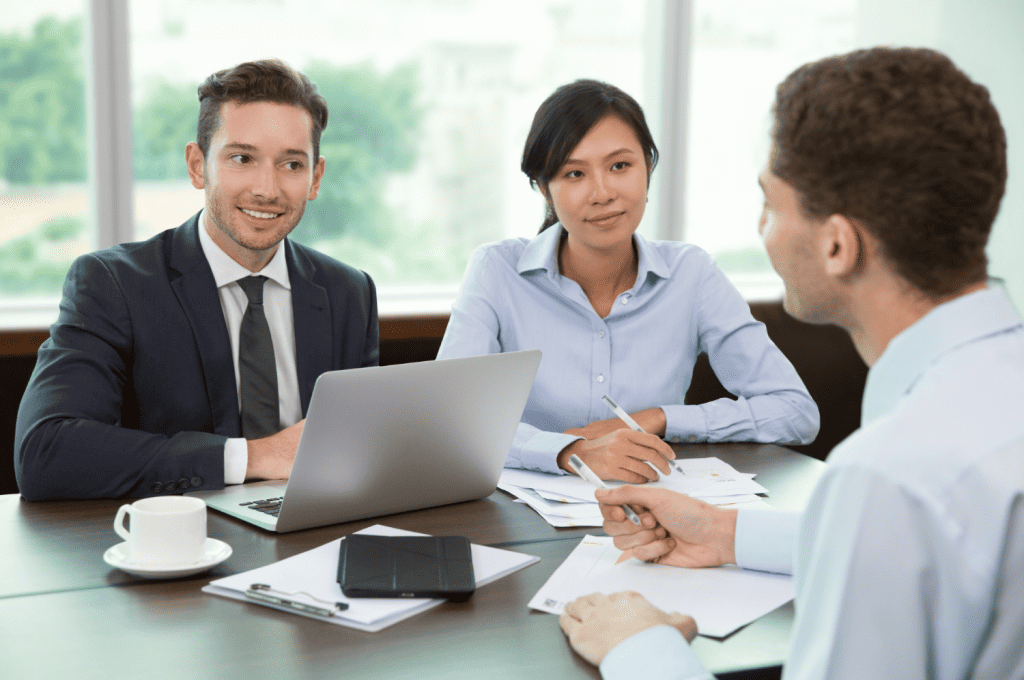
In this comprehensive guide, we will explore the foundations of predictive analytics, the intricacies of building effective retention models, and the ethical considerations surrounding employee data. Through case studies and success stories, we will showcase how leading organizations have harnessed the power of predictive analytics to reduce turnover and enhance employee satisfaction. Moreover, we will examine emerging trends and innovations that promise to shape the future of HR analytics.
Hirebee ensures that you are able to find the best candidates in the market for your team through industry-leading candidate screening as well as candidate sourcing features. Join us on this journey to unlock the potential of predictive analytics in preserving your organization’s most valuable asset—its people.
Table of Contents
ToggleUnderstanding Employee Turnover
Employee turnover is a critical phenomenon in the realm of human resources, and understanding its nuances is essential for crafting effective retention strategies. This section delves into the various aspects of employee turnover:
Definition and Types of Employee Turnover
Employee turnover refers to the rate at which employees leave an organization and are replaced by new hires. It is a multifaceted concept with different forms:
- Voluntary Turnover: Occurs when employees willingly leave their positions. Reasons for voluntary turnover may include career advancement opportunities, dissatisfaction with the current job, or personal reasons.
- Involuntary Turnover: Involves employees leaving the organization due to factors beyond their control, such as layoffs, company closures, or termination for performance issues.
- Functional Turnover: Happens when low-performing or disengaged employees voluntarily leave the organization, potentially improving overall workforce quality.
- Dysfunctional Turnover: Occurs when high-performing employees voluntarily depart, which can negatively impact the organization’s performance and morale.
The Impact of Turnover on Organizations
High employee turnover can have far-reaching consequences for businesses:
- Financial Implications: The cost of recruiting, hiring, and training new employees can be substantial. Moreover, the loss of knowledge and experience can hinder productivity and innovation.
- Decreased Morale: Frequent turnover can erode employee morale and lead to decreased job satisfaction among remaining staff.
- Disruption of Operations: Turnover disrupts workflow and can lead to a decrease in productivity as new employees adjust to their roles.
Common Reasons for Employee Turnover
Several factors contribute to employees deciding to leave an organization:
- Lack of Career Growth: Employees seek opportunities for career advancement and skill development. If these opportunities are not available, they may look elsewhere.
- Compensation and Benefits: Inadequate compensation or benefits packages can drive employees to seek better financial rewards elsewhere.
- Work-Life Balance: Striking a balance between work and personal life is crucial. Excessive workloads or inflexible schedules can prompt employees to leave.
- Poor Management and Leadership: Employees often cite issues with managers and leaders as a primary reason for departure. A toxic work culture or lack of trust in leadership can lead to turnover.
The Cost of Turnover
Calculating the true cost of turnover involves accounting for recruitment, training, decreased productivity, and potential lost business opportunities. Understanding these costs is vital for organizations seeking to address turnover through predictive analytics and targeted retention strategies.
The Basics of Predictive Analytics
Predictive analytics is a powerful approach that utilizes historical data, statistical algorithms, and machine learning techniques to make predictions about future events or outcomes. In the context of employee retention, it plays a pivotal role in forecasting and preventing turnover by identifying potential turnover risks before they manifest.
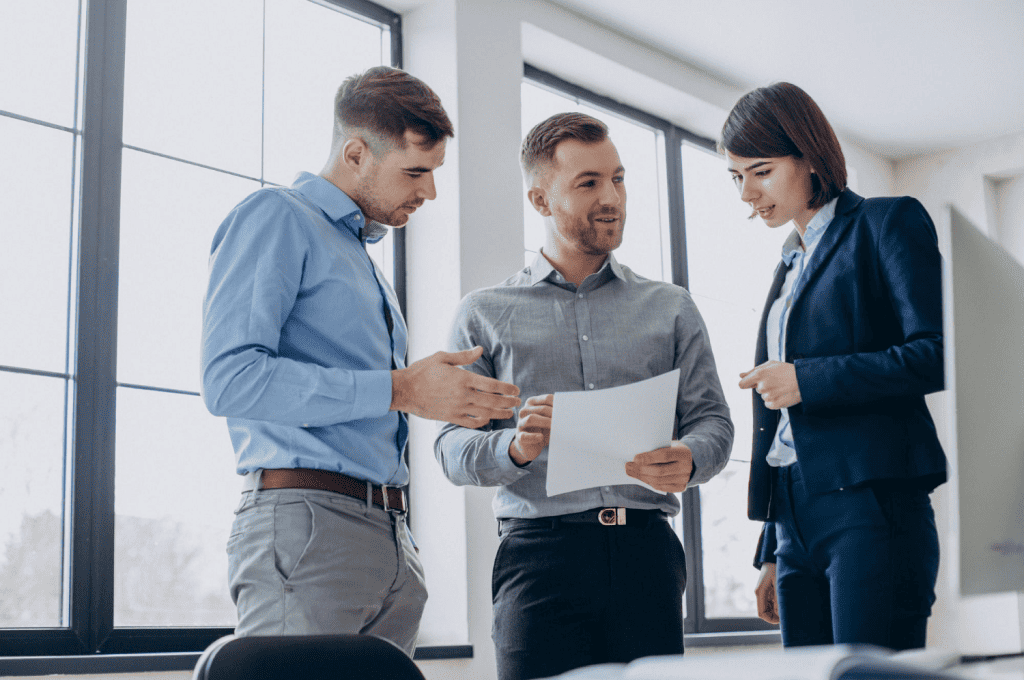
This section delves into the fundamental concepts and processes of predictive analytics:
Definition and Significance of Predictive Analytics:
Predictive analytics is the process of using data, statistical algorithms, and machine learning models to make predictions about future outcomes. In the realm of employee retention, it enables organizations to proactively identify employees who may be at risk of leaving and take preemptive actions to retain them. This not only saves costs associated with turnover but also fosters a more stable and productive workforce.
Data Sources for Predictive Analytics in Employee Retention:
Predictive analytics relies on historical data, which can be sourced from various HR systems, such as employee databases, time-tracking systems, performance evaluations, and employee engagement surveys. These data sources provide the raw material for building predictive models.
Key Metrics and Data Points to Consider:
To effectively predict employee turnover, organizations must consider a range of key metrics and data points. These may include but are not limited to employee demographics, job tenure, job performance, job satisfaction, compensation, and any previous instances of turnover. Identifying relevant data points is crucial for accurate model development.
Tools and Technologies for Predictive Analytics:
Organizations employ various tools and technologies for predictive analytics, including statistical software, programming languages (e.g., Python and R), and machine learning frameworks (e.g., TensorFlow and scikit-learn). Additionally, specialized HR analytics software and platforms have emerged to simplify the process of building and deploying predictive models in the HR context.
In summary, understanding the basics of predictive analytics is the foundation for effectively leveraging data to forecast and prevent employee turnover, ultimately leading to more informed HR decisions and improved employee retention rates.
Building a Predictive Analytics Model
Predictive analytics is a powerful tool for forecasting and preventing employee turnover, but its effectiveness hinges on constructing a robust model. This section outlines the essential steps in building a predictive analytics model for employee retention.
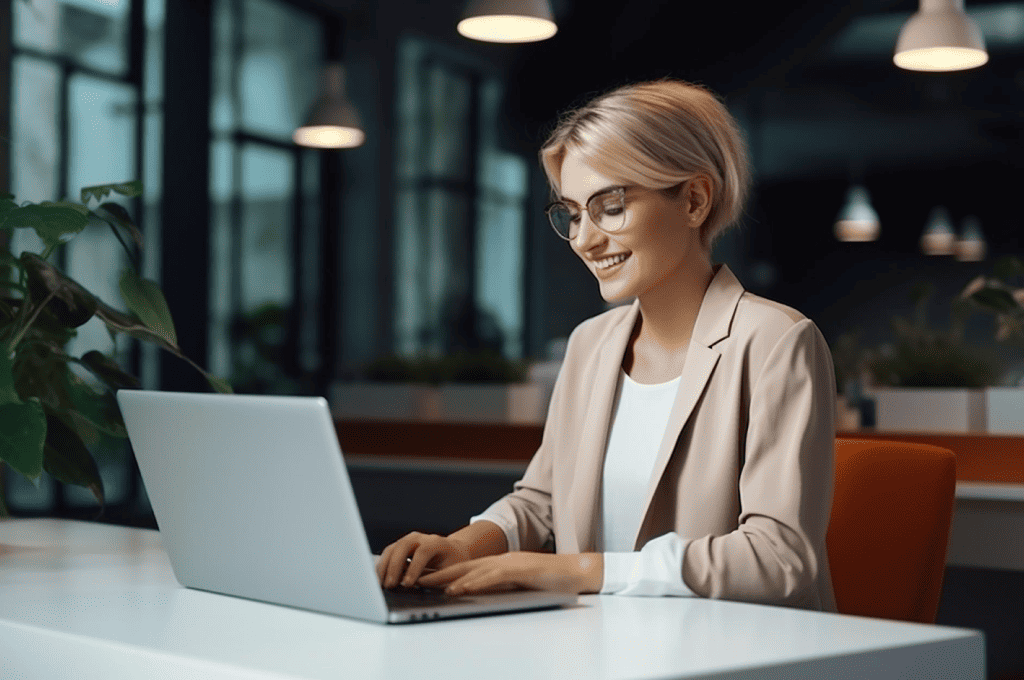
Firstly, data collection and preprocessing are critical. Organizations should gather relevant employee data from various sources, such as HR records, performance evaluations, and even external data like industry benchmarks. Ensuring data cleanliness and organization is vital to eliminate errors and inconsistencies.
Feature selection and engineering come next. Identifying the most pertinent features, such as job tenure, job satisfaction, and performance metrics, is essential. Additionally, creating new features or aggregating existing ones can provide valuable insights, like tenure-to-promotion ratios or engagement scores.
Choosing the right predictive model is a crucial decision. Regression models can predict turnover probabilities, while classification models classify employees into risk categories. The choice should align with the organization’s goals and the nature of its data.
Model training and validation involve splitting the data into training and testing sets to ensure the model’s generalizability. Cross-validation techniques further validate the model’s performance. Fine-tuning model parameters enhances accuracy.
Model evaluation metrics, such as accuracy, precision, recall, and F1-score, help assess the model’s effectiveness. ROC curves and AUC-ROC analysis provide insights into its discriminative power. Model interpretation and transparency ensure that the predictions are understandable and actionable.
Building a predictive analytics model requires careful planning and execution, but when done correctly, it equips organizations with the tools to proactively address employee turnover, fostering a more stable and engaged workforce.
Vaccination is a crucial component of public health, playing a vital role in preventing and controlling the spread of infectious diseases. It involves administering a weakened or inactivated form of a pathogen or a fragment of it, prompting the body’s immune system to produce antibodies without causing the disease itself. Here are some key points about vaccination:
1. Disease Prevention: Vaccination is a powerful tool for preventing a wide range of diseases, from childhood illnesses like measles, mumps, and polio to more recent threats like COVID-19. It helps establish herd immunity, reducing the overall prevalence of diseases in the community.
2. Lifesaver: Vaccines have saved countless lives throughout history. They have drastically reduced mortality rates from once-deadly diseases, such as smallpox, which was eradicated through vaccination efforts.
3. Safety: Vaccines go through rigorous testing and monitoring to ensure their safety and effectiveness. Adverse reactions are rare and often mild compared to the risks posed by the diseases they prevent.
4. Public Health Impact: Widespread vaccination programs have led to the near-elimination of some diseases, proving the effectiveness of immunization campaigns in promoting global health.
5. Continuing Research: Vaccination research remains ongoing to develop new vaccines, improve existing ones, and address emerging health threats.
6. Controversies: Despite their proven benefits, vaccines sometimes face skepticism and misinformation. Educating the public about the science and safety behind vaccines is crucial to maintain high vaccination rates and protect public health.
In summary, vaccination is a cornerstone of public health, preventing diseases, saving lives, and contributing to healthier communities worldwide. It underscores the importance of scientific research, public awareness, and global collaboration in safeguarding our health.
Data Privacy and Ethical Considerations
In the realm of predictive analytics for employee retention, data privacy and ethical considerations are paramount. Safeguarding employee data while harnessing its potential for predicting turnover is essential to maintain trust and uphold legal standards.
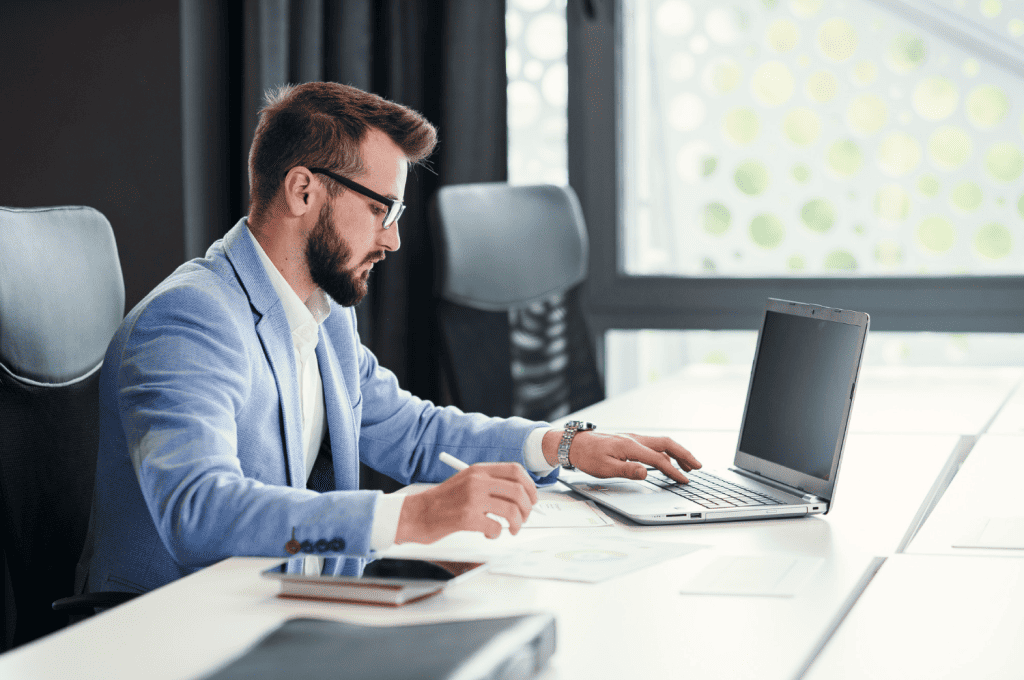
- Ensuring Data Security and Compliance: Organizations must adhere to stringent data protection regulations, such as GDPR or CCPA, when collecting and processing employee data. Implementing robust security measures, encryption protocols, and access controls are vital to prevent data breaches. It is crucial to inform employees about data usage and obtain their consent.
- Addressing Bias and Fairness: Predictive models can inadvertently perpetuate biases present in historical data. Ethical considerations dictate the need to identify and mitigate biases to ensure fairness in predictions. Regular audits and bias-mitigation techniques, such as re-weighting data or using fairness-aware algorithms, should be employed.
- Transparency and Accountability: Transparency is fundamental in maintaining trust. Employees should be informed about the use of predictive analytics in retention efforts and how it affects them. Furthermore, organizations should establish clear accountability structures, ensuring that decisions based on predictive analytics can be explained and reviewed.
Balancing the potential benefits of predictive analytics with ethical responsibilities is an ongoing challenge. Striking the right equilibrium is essential to not only harness the power of these tools but also to foster a culture of trust and transparency within the organization. Ultimately, responsible and ethical use of predictive analytics can enhance employee retention efforts while preserving the rights and dignity of individuals.
Conclusion
In conclusion, predictive analytics has emerged as a powerful tool in the realm of employee retention. By harnessing the potential of data and machine learning, organizations can identify at-risk employees, forecast turnover probabilities, and tailor retention strategies effectively. While challenges such as data quality and ethical considerations persist, the benefits of predictive analytics in reducing turnover and enhancing workforce stability are undeniable. As the landscape of HR continues to evolve, embracing these innovative techniques becomes essential for organizations seeking to retain talent and remain competitive. The future holds exciting prospects, promising further advancements in predictive analytics for employee retention.
FAQs
What is predictive analytics in the context of employee retention?
Predictive analytics in employee retention involves using data and statistical models to forecast which employees are at risk of leaving the organization, allowing proactive measures to be taken to prevent turnover.
Why is employee retention important for organizations?
Employee retention is crucial for maintaining a stable workforce, reducing recruitment costs, preserving institutional knowledge, and fostering a positive work culture. High turnover can be costly and disruptive.
What types of data are used for predictive analytics in employee retention?
Employee data such as performance metrics, job tenure, salary, engagement surveys, and demographic information are commonly used. Other factors like work-life balance and commute time can also be considered.
How accurate are predictive analytics models for employee retention?
The accuracy of predictive models varies depending on data quality and model sophistication. When properly trained and validated, these models can provide valuable insights into turnover risk.
What steps can organizations take to use predictive analytics for employee retention effectively?
Organizations should collect and clean relevant data, choose appropriate predictive models, and invest in employee training to interpret and act on the results.