The field of recruitment has undergone a transformative shift with the advent of predictive analytics. In today’s competitive job market, organizations are increasingly turning to data-driven strategies to gain a competitive edge in talent acquisition. This paper delves into the critical role of predictive analytics in recruitment, focusing on its ability to forecast time to hire and optimize pipeline efficiency.
Predictive analytics harnesses historical data and advanced modeling techniques to provide insights into the hiring process, enabling organizations to anticipate the time required to fill vacant positions and streamline their candidate pipelines. By exploring the theoretical foundations, methodology, and real-world applications, this paper aims to underscore the significance of predictive analytics as a strategic tool for modern recruitment practices. As the business landscape continues to evolve, understanding and harnessing the power of predictive analytics can lead to more informed, efficient, and successful recruitment endeavors.
Table of Contents
ToggleTheoretical Framework
Predictive analytics has emerged as a transformative approach in modern recruitment practices, leveraging data-driven insights to enhance decision-making. This section delves into the fundamental concepts underpinning predictive analytics in recruitment, offering a theoretical foundation for the subsequent exploration of forecasting time to hire and optimizing pipeline efficiency.
Predictive analytics involves the application of statistical algorithms, machine learning techniques, and data mining to predict future outcomes based on historical and current data. In the context of recruitment, it enables organizations to anticipate and streamline critical aspects of the hiring process. Key concepts such as feature engineering, model selection, validation strategies, and evaluation metrics provide the tools necessary for constructing accurate predictive models.
This framework highlights the potential benefits of incorporating predictive analytics into recruitment efforts. By analyzing historical hiring data, organizations can gain actionable insights into candidate behavior, recruitment bottlenecks, and factors influencing hiring timelines. Furthermore, predictive analytics empowers recruiters to proactively address challenges by identifying high-potential candidates and optimizing the candidate pipeline.
Overall, this theoretical groundwork establishes the rationale for harnessing predictive analytics in the realm of recruitment. It sets the stage for a comprehensive exploration of how these concepts can be effectively employed to forecast time to hire and enhance pipeline efficiency, ultimately leading to more informed and efficient hiring decisions.
Forecasting Time to Hire
Forecasting the time to hire is a crucial aspect of recruitment management, enabling organizations to streamline their hiring processes and make informed decisions. Time to hire, defined as the duration between a candidate’s initial application and their final acceptance of a job offer, serves as a vital metric for assessing recruitment efficiency. Various factors influence this metric, including the complexity of the job role, the effectiveness of candidate sourcing channels, and the efficiency of interview and assessment processes.
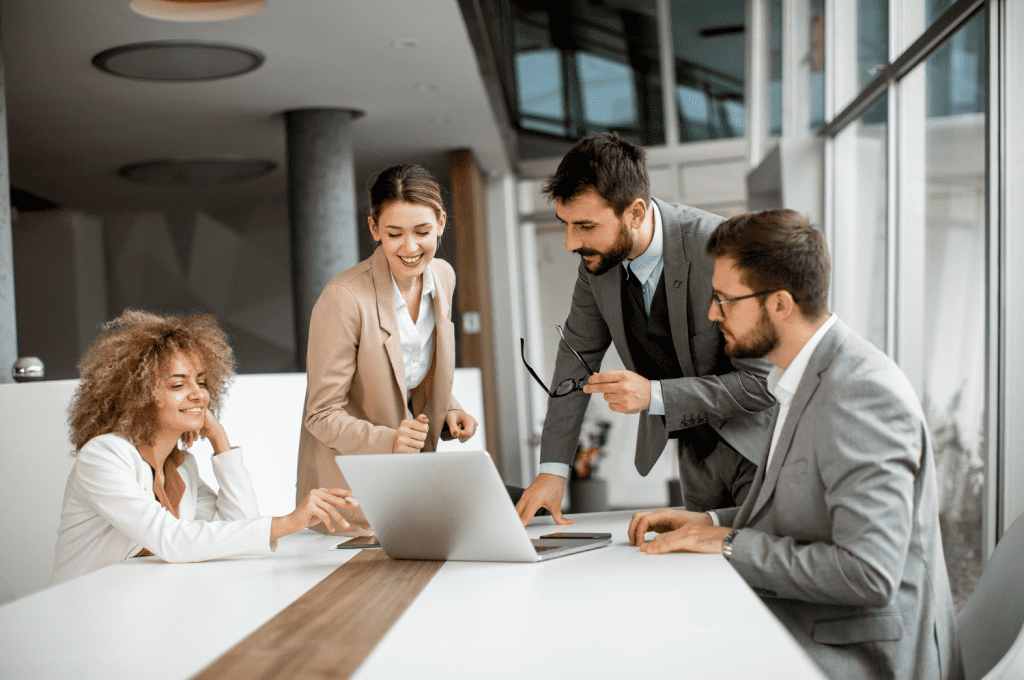
To accurately forecast time to hire, a systematic approach is required. Data collection and preprocessing involve gathering historical recruitment data, cleaning and organizing it, and identifying relevant variables for analysis. Subsequently, suitable predictive modeling techniques must be selected and developed, ranging from traditional regression models to advanced machine learning algorithms like Random Forest and XGBoost. The process of feature engineering and selection is essential to ensure that the chosen model captures the most influential factors affecting time to hire.
Model validation and evaluation metrics are employed to assess the predictive accuracy of the developed model. Techniques such as cross-validation and root mean square error (RMSE) provide insights into how well the model performs on unseen data. Interpretation of the results yields actionable insights for recruitment managers, allowing them to identify bottlenecks in the hiring process, allocate resources effectively, and optimize various stages of recruitment. By employing predictive analytics to forecast time to hire, organizations can enhance their recruitment strategies and make timely, data-driven decisions to attract and retain top talent.
Optimizing Pipeline Efficiency
Efficient candidate pipeline management is essential for organizations seeking to enhance their recruitment processes. This section focuses on strategies to optimize pipeline efficiency through the application of predictive analytics.
Firstly, defining pipeline efficiency involves understanding the various stages candidates go through, from sourcing to hiring, and identifying potential bottlenecks or areas of improvement. Challenges such as candidate drop-offs, delays in decision-making, and inadequate communication can hinder pipeline flow.
To address these challenges, data integration and aggregation play a crucial role. By consolidating data from various sources, including applicant tracking systems and recruitment platforms, organizations can create a comprehensive view of their pipeline. This enables the development of predictive models that analyze historical data to forecast candidate progression and potential hurdles.
Predictive modeling techniques, including classification models and clustering algorithms, contribute to effective pipeline optimization. Classification models predict the likelihood of candidates progressing to the next stage, aiding recruiters in allocating resources to high-potential candidates. Clustering techniques segment candidates based on shared characteristics, enabling tailored communication and engagement strategies.
Incorporating external factors, such as market trends and economic conditions, further refines pipeline optimization. Adapting strategies based on these factors enhances the accuracy of predictions and enables proactive decision-making.
Moreover, strategies like targeted candidate engagement and predictive nurturing help maintain an active and engaged talent pool. By leveraging predictive insights, recruiters can personalize communication, recommend relevant job opportunities, and mitigate the risk of losing promising candidates to competitive offers.
Measuring the impact of pipeline optimization involves quantifying improvements in key performance metrics, such as time to hire, conversion rates, and candidate satisfaction. The successful implementation of predictive analytics in pipeline management leads to streamlined processes, reduced time-to-fill, and enhanced candidate experiences.
In conclusion, leveraging predictive analytics for optimizing pipeline efficiency empowers organizations to make informed decisions, streamline recruitment processes, and build stronger relationships with potential candidates. By addressing challenges, incorporating external factors, and implementing tailored strategies, predictive analytics becomes a valuable tool in creating a more effective and agile recruitment pipeline.
Case Study: Application of Predictive Analytics
In this section, we delve into a practical application of predictive analytics in the realm of recruitment. We select a real-world recruitment scenario to showcase the efficacy of forecasting time to hire and optimizing pipeline efficiency using data-driven approaches.
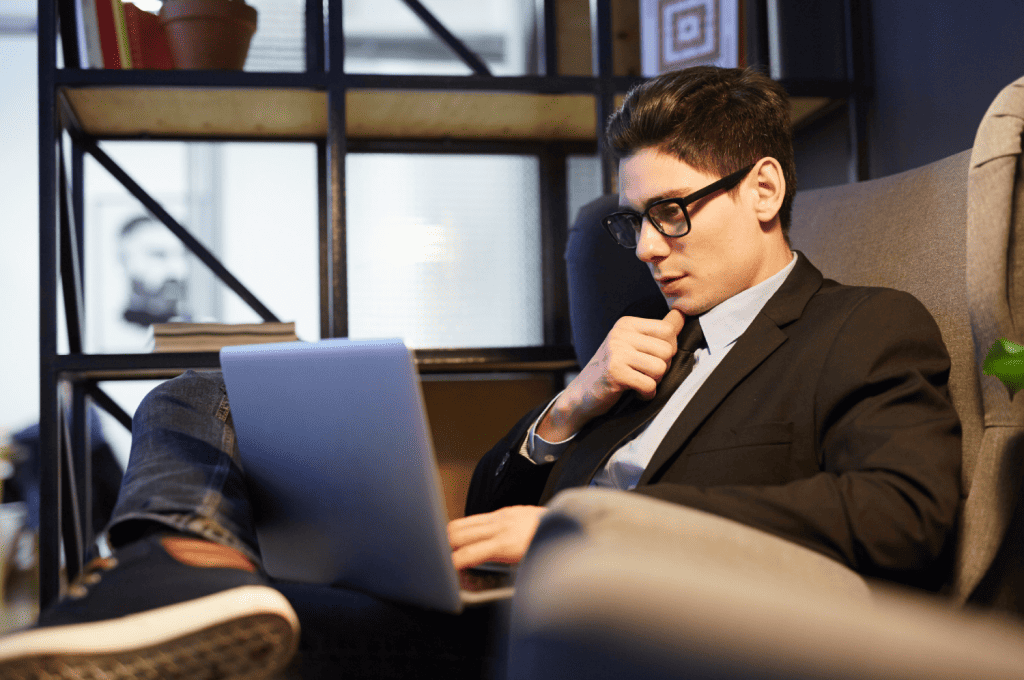
Selection of a Real-World Recruitment Scenario
We carefully choose a specific job role or position within an organization to serve as the focal point of our case study. This choice is guided by factors such as the job’s historical data availability, its significance to the organization, and its potential impact on business operations.
Data Collection, Preprocessing, and Preparation
Detailed insights are provided into the data collection process, emphasizing the importance of gathering comprehensive and accurate data. We discuss the challenges associated with data quality, integration, and formatting. Preprocessing steps, including data cleaning, transformation, and feature engineering, are explained to ensure the dataset’s suitability for modeling.
Model Development for Time to Hire Forecasting
We outline the steps involved in building a predictive model for forecasting time to hire. This encompasses the selection of appropriate algorithms, model training, cross-validation techniques, and hyperparameter tuning. The integration of relevant features, such as candidate qualifications, sourcing channels, and interview stages, is elucidated.
Model Development for Pipeline Efficiency Optimization
Our focus shifts to developing a model aimed at optimizing the recruitment pipeline’s efficiency. We explore classification models to predict candidate progression and clustering techniques for segmenting candidates based on their likelihood of success. The inclusion of external factors, such as market trends and economic indicators, enhances the model’s predictive capabilities.
Implementation Challenges and Solutions
The practical challenges encountered during the implementation of predictive analytics in recruitment are candidly discussed. Issues such as data silos, model interpretability, and change management are addressed, along with strategies employed to overcome these hurdles.
Results and Insights Gained from the Case Study
The section culminates with a presentation of the results obtained from the case study. We showcase the accuracy of time to hire predictions and the tangible improvements achieved in pipeline efficiency. The insights gleaned from the models’ outputs, including identification of bottlenecks and opportunities for proactive candidate engagement, are highlighted.
Incorporating this real-world case study provides a tangible illustration of the power of predictive analytics in revolutionizing recruitment practices. It underscores the practical relevance of forecasting time to hire and optimizing pipeline efficiency, paving the way for data-driven decision-making in talent acquisition.
Ethical Considerations
Predictive analytics in recruitment offers promising opportunities, but its application raises important ethical considerations that demand careful attention. As organizations harness data-driven insights to optimize their hiring processes, several ethical challenges emerge that must be addressed to ensure fairness, transparency, and accountability.
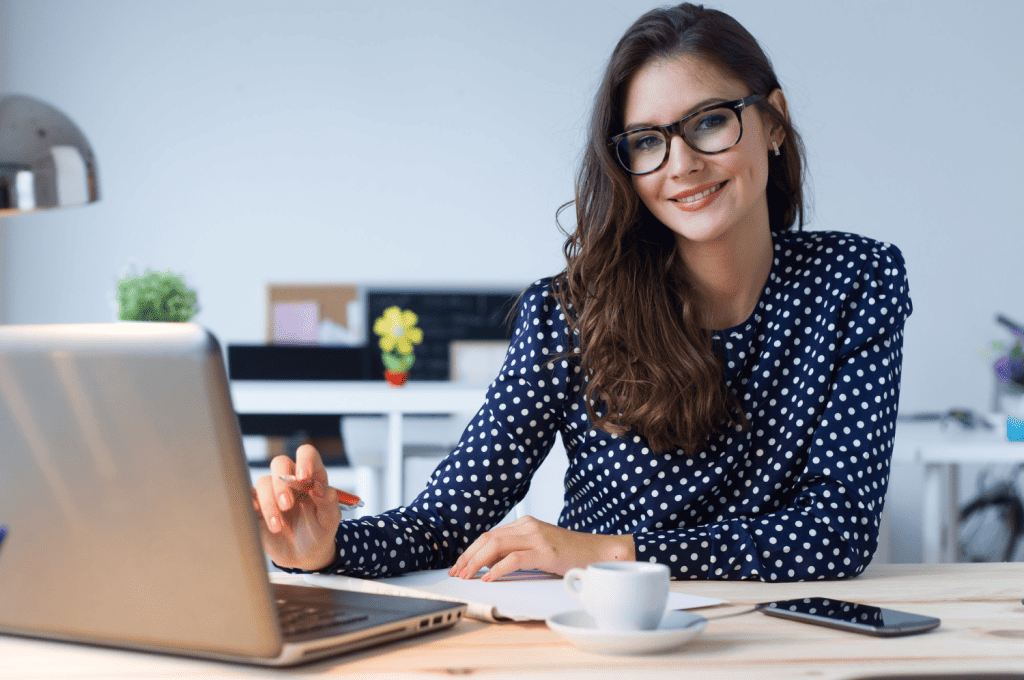
Addressing Bias and Fairness Issues
Predictive models can inadvertently perpetuate bias if historical data reflects discriminatory practices. It is imperative to meticulously examine training data for biases related to race, gender, and other protected characteristics. Mitigation strategies, such as reweighting data, using fairness-aware algorithms, and regular audits, must be employed to rectify and prevent biased outcomes. An ongoing commitment to diversity and inclusion is essential to mitigate bias and create a more equitable hiring process.
Privacy Concerns and Data Security
Utilizing extensive candidate data for predictive analytics necessitates stringent data privacy measures. Organizations must adhere to data protection regulations, obtain informed consent, and ensure data security throughout the entire analytics pipeline. Anonymization and aggregation techniques can help balance data utility with individual privacy, assuaging concerns about sensitive information exposure.
Transparency and Accountability
The opacity of predictive models can erode trust between candidates and employers. Transparent model explanations and interpretations should be provided to candidates when decisions are influenced by predictive analytics. Organizations must also establish mechanisms for candidates to appeal decisions and seek recourse in case of errors or disputes.
Incorporating these ethical considerations into the implementation of predictive analytics in recruitment not only aligns with societal expectations but also enhances the overall credibility and reliability of the hiring process. Striking a balance between leveraging data-driven insights and upholding ethical standards is pivotal to realizing the full potential of predictive analytics while maintaining a fair and just recruitment ecosystem.
Conclusion
In conclusion, predictive analytics offers a transformative approach to recruitment by forecasting time to hire and enhancing pipeline efficiency. Through data-driven insights, organizations can streamline their hiring processes, reduce time-to-fill, and make informed decisions. However, ethical considerations like bias mitigation and data privacy must remain paramount. As the field evolves, embracing emerging technologies and methodologies will be crucial. By harnessing the power of predictive analytics responsibly, recruiters can create a more equitable, efficient, and competitive hiring landscape, ultimately driving better outcomes for both candidates and businesses.
FAQs
What is predictive analytics in the context of recruitment?
Predictive analytics in recruitment involves using historical and real-time data to develop models that forecast various aspects of the hiring process. These models can predict the time it takes to hire a candidate, optimize the efficiency of the recruitment pipeline, and provide insights into candidate progression.
Why is forecasting time to hire important for recruitment?
Forecasting time to hire helps organizations streamline their recruitment processes by providing insights into when candidates are likely to be hired. This allows for better resource allocation, reduced time and cost-to-fill positions, and improved workforce planning.
How does predictive analytics optimize the recruitment pipeline efficiency?
Predictive analytics optimizes the recruitment pipeline by identifying potential bottlenecks and inefficiencies. It helps in segmenting and prioritizing candidates, enabling recruiters to focus efforts where they are most likely to yield successful hires. This leads to reduced candidate drop-offs, improved candidate experience, and faster overall hiring timelines.
What factors are considered in predicting time to hire?
Factors influencing time to hire include the complexity of the job role, the sourcing channels used to attract candidates, the efficiency of interview and assessment processes, and external factors like market demand and economic conditions.
How are predictive models developed for recruitment analytics?
Predictive models are developed through data collection, preprocessing, and selection of appropriate algorithms such as regression models or machine learning techniques like Random Forest or XGBoost. Feature engineering and model validation are crucial steps to ensure accurate predictions.